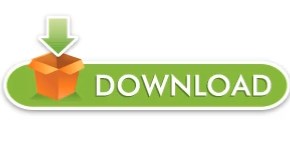
- #Best open source software for mac how to#
- #Best open source software for mac mac os#
- #Best open source software for mac software#
- #Best open source software for mac license#
Its applications are basically data-mining and any other machine learning task where decision trees could be useful. SMILES extends classical decision tree learners with new splitting criteria, new partitions, non-greedy search, extraction of several and different solutions.
#Best open source software for mac software#
SMILES is an open source machine learning software system that incorporates different features from other machine learning techniques and paradigms and, more importantly, it presents several innovations in almost all of these features.
#Best open source software for mac mac os#
Orange works on Windows, Linux, and Mac OS X. When it comes to decision trees, Orange has numerous implementations of classification tree learners: TreeLearner, SimpleTreeLearner, and a C45Learner. In addition, Orange graphic user interface allows you to focus on exploratory data analysis instead of coding. Orange is used for teaching at schools, universities and in professional training courses all over the world. It allows you to explore statistical distributions, box and whisker plots, scatter plots, or dive much deeper with decision trees, heatmaps, MDS, hierarchical clustering, and linear regression models. Orange is a free and open source data visualization software and machine learning tool for novice and expert. The software works on Windows, Linux, and Mac OS X. In addition, they will provide you with a rich set of examples of decision trees in different areas such as research and development project decision tree, city council management software and etc. The decision tree can be easily exported to JSON, PNG or SVG format. SilverDecisions is a free and open source decision tree software with a great set of layout options. It is a specialized software for creating and analyzing decision trees.

Rapid Miners runs on Windows, Linux, and Mac OSX. Written in Java, it holds a variety of data mining functions such as visualization, data pre-processing, cleansing, filtering, clustering, and predictive analysis. Its Decision Tree operator generates a decision tree model, which can be used for classification and regression. And it is one of the best open source decision tree software tool with no-coding required. This is a very powerful and popular data mining software solution which provides you with predictive advanced analytics. Knime is available for Windows, Linux, and Mac OSX.
#Best open source software for mac how to#
In addition, KNIME has great examples of how to build prediction or classification model using a decision tree algorithm.
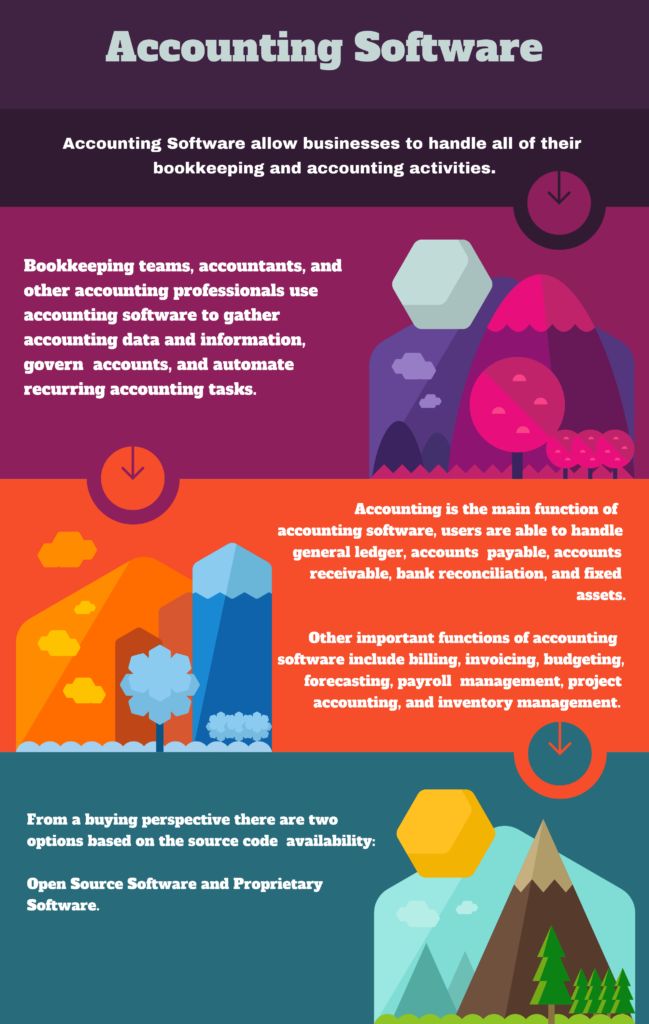
KNIME will provide you with 1500 modules, hundreds of ready-to-run examples (including decision tree examples), a variety of integrated tools, and an extremely wide choice of advanced algorithms. The platform is fast to deploy, easy to scale, and very intuitive to learn. KNIME Analytics Platform is one of the best open solutions for data-driven innovation. Moreover, Weka has free online courses that teach machine learning and data mining using Weka. Weka contains tools for classification, regression, visualization and clustering, and association rules. The algorithms can be applied directly to a dataset as well as called from Java code.

This is a Java-based free and open source tool for Windows, Linux, and Mac OS X. Weka is a powerful collection of machine learning algorithms for data mining purposes.
#Best open source software for mac license#
On this page, we collected 10 best open source license classification tree software solutions that run on Windows, Linux, and Mac OS X.

Over the past few years, open source decision tree software tools have been in high demand for solving analytics and predictive data mining problems.ĭecision trees are a popular type of supervised learning algorithm that builds classification or regression models in the shape of a tree (that’s why they are also known as regression and classification trees). They work for both categorical data and continuous data.
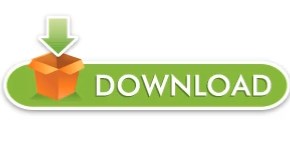